Introduction
An acute respiratory syndrome (COVID-19), caused by a novel coronavirus (SARS-CoV-2), has spread from China worldwide and caused a pandemic that brought severe medical, social, and economic problems. The COVID-19 infection results in various clinical presentations ranging from an asymptomatic course and mild flu-like clinics to severe pneumonia and hyperinflammatory response, potentially requiring intensive care and even causing death. In severe cases, COVID-19 is a systemic disease with hyper-inflammation, cytokine storm, and elevated cardiac enzymes (1-3).
Recently, several studies have tried explaining the pathways and incomplete links between the humoral and cellular immune systems and COVID-19 (4-7). There are several risk factors associated with mortality in COVID-19 patients, notably age, hypertension, diabetes mellitus (DM), cardiovascular disease, D-dimer, neutrophil count, lymphocyte count, neutrophil/lymphocyte ratio (NLR), C-reactive protein (CRP), and others (8, 9). It is also known that rapid risk classification and early medical intervention reduce mortality in COVID-19. Furthermore, several studies have investigated the prognostic factors in COVID-19, such as C-reactive protein, alanine aminotransferase (ALT), aspartate aminotransferase (AST), albumin, creatinine, creatine kinase, D-dimer, procalcitonin, ferritin, lactate dehydrogenase (LDH), neutrophil count, lymphocyte count, NLR, monocyte to lymphocyte ratio (MLR), platelet to lymphocyte ratio (PLR), and other inflammatory parameters (10-14). Thus, systemic inflammatory response markers are reasonably used in the clinical follow-up of COVID-19 patients. However, few studies have used inflammation-based risk scores such as the Glasgow Prognostic Score (GPS), Prognostic Index (PI), Prognostic Nutrition Index (PNI), and Systemic Inflammatory Index (SII) to evaluate the mortality risk in hospitalized patients with COVID-19. Therefore, we evaluated all four inflammation-based prognostic scores in our study.
In the literature, GPS was previously associated with inflammatory processes such as cancer and acute myocardial infarction (15, 16). Besides, PI, consisting of serum CRP and peripheral white blood cell (WBC) count, was reported to be a strong predictor of survival in advanced lung cancer patients (17). On the other hand, PNI, calculated according to serum albumin and peripheral lymphocyte count, was reported as an independent predictor of poor outcomes in patients with hepatocellular carcinoma (18). SII, defined as the platelet count x neutrophil count/lymphocyte count, had a high prognostic value in cancer patients (19).
In this study, we aimed to investigate the predictive value of inflammation-based risk scores, including GPS, PNI, PI, and SII, on in-hospital mortality due to COVID-19, defined as a complex inflammatory disease related to immune deficiency.
Materials and Methods
Study Design and Participants
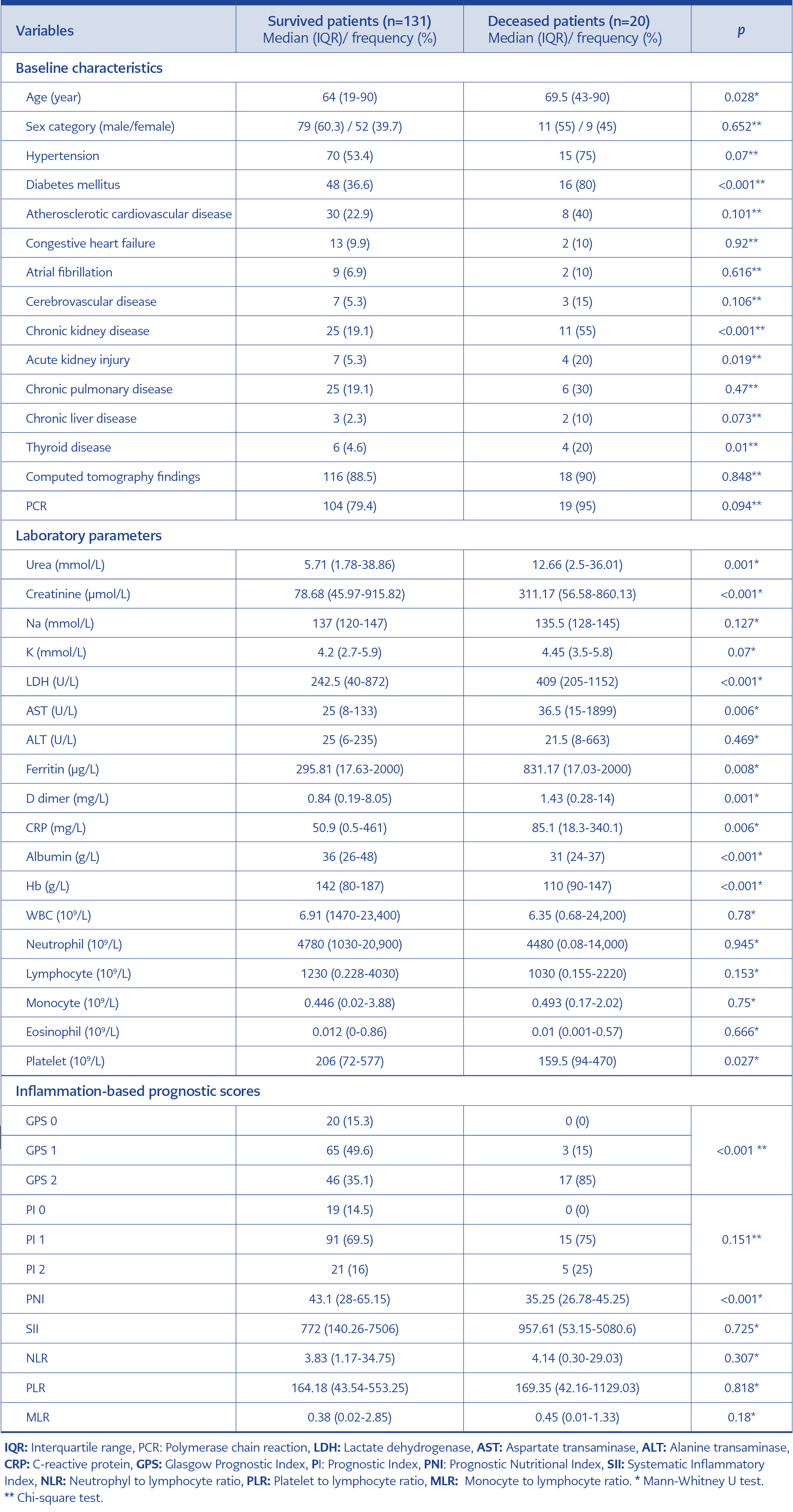
Table 1. Comparison of the baseline demographics and clinical characteristics between survived and deceased patients.
Three hundred thirty-six patients older than 18 years of age with a diagnosis of COVID-19 confirmed by only PCR testing or additional chest tomography between March 2020 and August 2020 were enrolled in this retrospective cross-sectional study. Patients with missing values of serum CRP, creatinine, albumin, platelet count, and lymphocyte count, cancer patients, patients using immunosuppressive therapy for transplantation, and patients with autoimmune disease were excluded. The treatment protocols of the COVID-19 patients were tailored by the hospital’s infectious diseases and chest diseases departments. Among 336 patients, 159 (47.3%) had missing data, seven (2%) were referred to another hospital at the time of diagnosis, seven (2%) had cancer, seven (2%) were renal transplant patients, three (0.8%) were liver transplant patients, and two (0.6 %) were patients treated for the rheumatologic diseases. Totally 151 patients followed up in the intensive care unit were found eligible for the study. The patient selection flowchart is shown in Figure 1. Deceased and survived COVID-19 patients’ data were analyzed separately in two groups.
This study complied with the Declaration of Helsinki, and the Başkent University Ethics Committee approved the study with decision number 22/112 on May 25, 2022. Also, the data used in the study were anonymous, so informed consent was not required.
Data Collection, Definitions, and Calculations
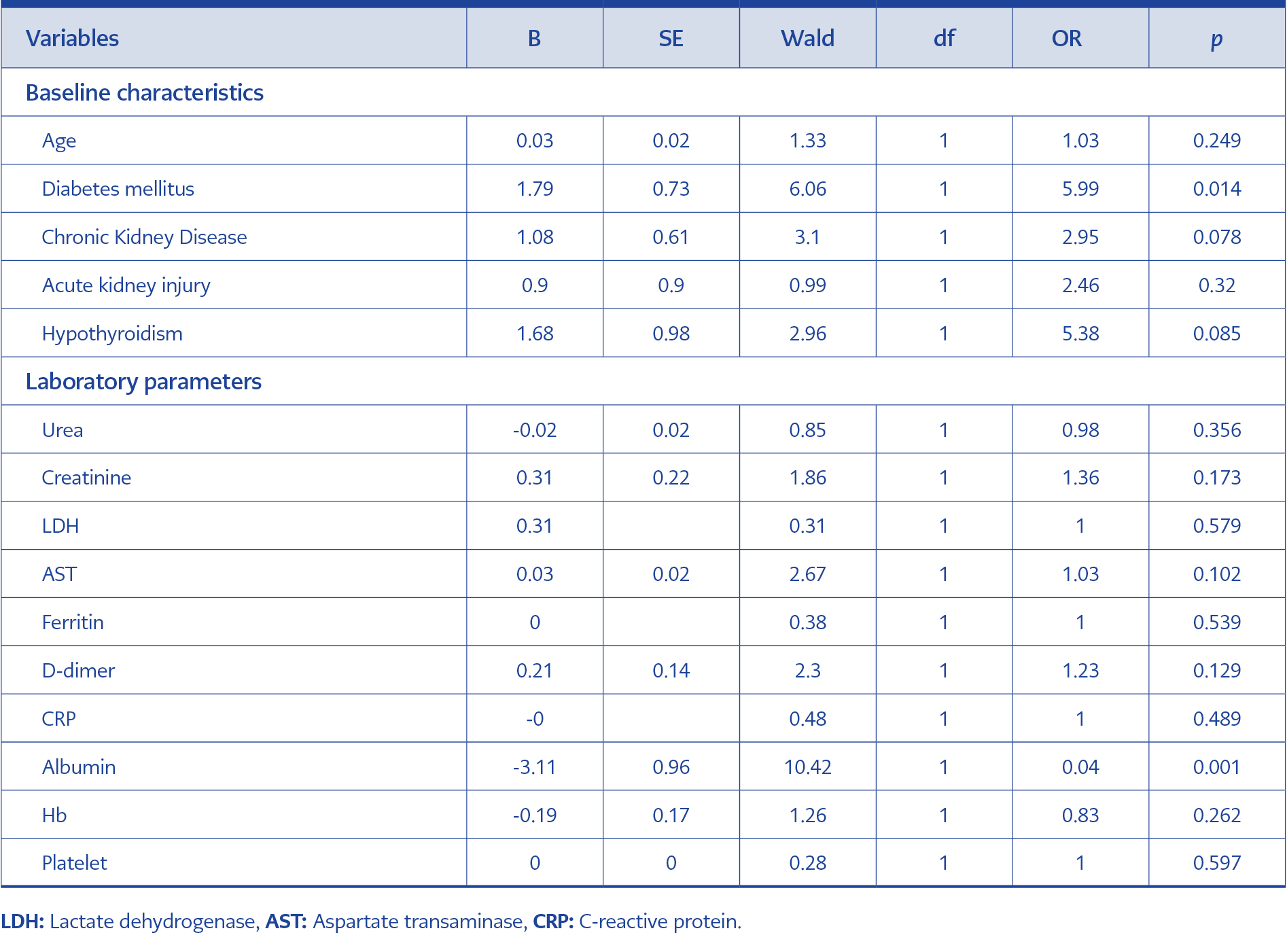
Table 2. Multivariable logistic regression analysis of the baseline characteristics and laboratory parameters.
All data, including demographic features, clinical characteristics, pre-existing comorbidities, the data required to calculate the prognostic scores of COVID-19 patients at admission, laboratory findings, chest-computed tomography results, and the duration of hospital stay, were obtained from the hospital’s data automation system and written medical records. Two researchers reviewed all data and calculations to confirm the accuracy. PNI, one of the inflammatory scoring systems, was calculated using serum albumin (g/L) + 5 x peripheral blood lymphocyte count (109/L). PI was calculated by scoring based on CRP and WBC count. GPS was calculated by scoring based on serum albumin and CRP. The SII, a new inflammatory biomarker, was calculated using blood platelet count x neutrophil count/lymphocyte count.
Statistical Analyses
The statistical analysis was performed using the Statistical Package for the Social Sciences (SPSS) 25.0 program (IBM Corp., Armonk, NY, USA). Histogram graphics and skewness kurtosis values were examined to investigate whether the data were normally distributed. Thus, descriptive statistics for non-parametric quantitative variables included median (interquartile range [IQR], min-max), and numbers and percentages for categorical variables. Mann-Whitney U test was used to compare non-normally distributed variables. Chi-square or Fischer’s exact test was used for categorical variables. Using univariate analysis, we tested the demographic, clinical, biochemical variables, and inflammation-based prognostic risk scores. The variables with a p<0.2 were put into binary logistic regression analysis, and independent predictors of mortality were sought. We applied the receiver operating characteristic (ROC) curve analysis and area under the ROC (AUC) to assess the predictive power of the independent predictors of in-hospital mortality. A p-value <0.05 was set as statistically significant for all tests. In addition, we used the G*Power software v.3.1.9.7 (Heinrich-Heine-Universität Düsseldorf, Düsseldorf, Germany) to assess the post hoc power of logistic regression analysis, although the post hoc power analysis used to indicate the strength of the results may be misleading and overfitting.
Results
Of 151 patients, 90 (59.6%) were male, and 61 (40.4%) were female. In the surviving patients (n=131), 79 (60.3%) were male, and 52 (39.7%) were female. The median age of the surviving patients was 64, whereas it was 69.5 in deceased patients. The polymerase chain reaction (PCR) test was positive in 123 patients, and 134 patients’ CT findings were typical. Furthermore, the overall in-hospital mortality rate was 13.2%.
In the univariate analyses, the baseline variables of age, DM, chronic kidney disease, acute kidney injury, hypothyroidism, LDH, AST, D-dimer, ferritin, CRP, albumin, hemoglobin level, platelet count, urea, creatinine level, PNI, GPS were significantly associated with mortality. In contrast, PI, SII, NLR, PLR, and MLR (monocyte to lymphocyte ratio) were not associated with mortality (Table 1). We also observed a relationship between the duration of hospital stay and mortality. The mean hospital stay was seven days (min=1-max=53) in survived COVID-19 patients, while it was 14.5 days (min=5-max=45) in deceased patients (p<0.001).
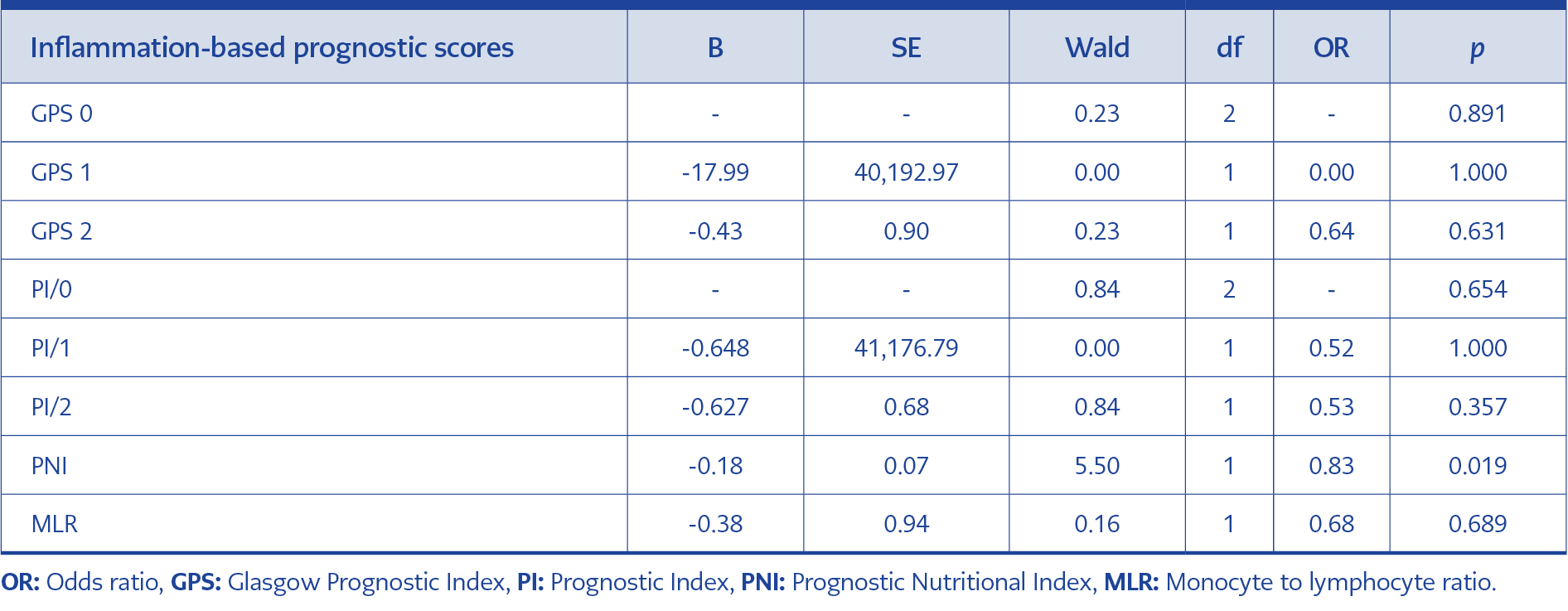
Table 3. Multivariable logistic regression analysis of the inflammation-based prognostic risk scores.
In binary logistic regression analysis of baseline characteristics and laboratory parameters, DM (OR=5.99; [95% CI=1.44-24.93]; p=0.014), the length of hospital stay (OR 1.10 [95% CI 1.04-1.16], p<0.001), and albumin (OR=0.045; [95% CI=0.01-0.29]; p=0.001) were found to predict in-hospital mortality (Table 2). Moreover, in the binary logistic regression analysis of the inflammation-based prognostic scores, only PNI was found to be statistically significant in predicting in-hospital mortality (OR=0.83; [95% CI 0.71-0.97]; p=0.019) (Table 3).
The regression analysis of inflammation-based variables predicting in-hospital mortality had a Hosmer-Lemeshow p-value of 0.945 and an explanatory coefficient Nagelkerke R2 value of 0.305, which indicated the goodness of fit of the regression model. Based on mean PNI score levels, the observed (post hoc) power was assessed using G*Power software v.3.1.9.7. Logistic regression analysis with two-tailed testing for an α=0.05 and a sample size of 151 revealed the power (1- β) of >0.99 with a critical z value of 1.64 in the G*Power software v.3.1.9.7. As PNI was the sole predictor among these inflammation-based prognostic risk scores for in-hospital mortality in binary logistic regression analysis, a ROC curve analysis for PNI was performed. The AUC of the PNI was 0.797 (95% CI=0.696-0,898; p<0.001) (Figure 2). At the 38.12 cutoff value, PNI had a sensitivity of 65% and a specificity of 71%, with a positive likelihood ratio of 2.24 and a negative likelihood ratio of 0.49. For an optimal cutoff value of 40.71, PNI had a sensitivity of 80.0% and a specificity of 64.1% for predicting in-hospital mortality, whereas the positive likelihood ratio was 2.22 and the negative likelihood ratio was 0.31.
We found that there was a significant difference between COVID-19 survivors and non-survivors in terms of age, DM, chronic kidney disease, acute kidney injury, hypothyroidism, length of hospital stay, LDH, AST, D-dimer, ferritin, CRP, albumin, hemoglobin level, platelet count, urea, creatinine level, GPS, and PNI. In addition, multiple logistic regression analysis indicated that the PNI had the best predictive value among inflammation-based prognostic risk scores (GPS, PI, PNI, and SII) for COVID-19 patients.
Discussion
Recent articles describing the possible mechanisms of COVID-19 causing disease and affecting immunity defined it as a complex systemic inflammatory disease (4-7). Many clinical features and laboratory parameters, including age, abnormal cellular and humoral immunity, neutrophilia, lymphocytopenia, low CD4+ T cells and C3 level, underlying diseases, secondary infection, and elevated inflammatory indicators, were assessed to predict mortality of patients with COVID-19. In several studies, advanced age, DM, WBC, neutrophil count, platelet count, CRP, D-dimer, ferritin, LDH, albumin, creatinine levels, interleukin-6 (IL-6), NLR were significantly associated with COVID-19 disease severity (7, 12-14, 20). In addition, poor nutritional status and immune dysfunction were considered risk factors for severe infection caused by SARS-CoV-2 (21).
Inflammation-based prognostic risk scores are available for malignant tumors such as digestive system malignancies, lung malignancies, chronic kidney diseases, or inflammatory diseases such as myocardial infarction. Among these inflammation-based risk scores, GPS, PI, PII, and SPII are predictive of mortality, especially in patients with malignancy. GPS reflects both inflammation and nutrition status. Besides, PNI, calculated from serum albumin concentration and lymphocyte count, was initially used in patients undergoing surgical treatment of digestive system diseases to evaluate the immunological and nutritional aspects. Similar to PNI and GPS, PI and SII are the other inflammation-based risk scores used in the prediction of disease progression and mortality in malignancies or other inflammatory diseases (22-24).
Several studies have been conducted on inflammatory parameters and COVID-19 disease severity, morbidity, and mortality. However, to our knowledge, there are few studies about PNI and other inflammation-based prognostic scores in COVID-19 (21, 25-27). In one of these studies, PNI and SII were independent predictors of the prognosis and the disease severity in COVID-19 patients requiring hospitalization at the intensive care unit. In that study, PNI had a 73.4% sensitivity and 70.8% specificity in predicting the severity of the disease and admission to the intensive care unit when the cutoff value was 36.7 (25). Another study showed that PNI was independently associated with COVID-19 and predicted the disease severity and prognosis with 85.7% sensitivity and 60% specificity when the cutoff value was 43 (26). In a recent systematic review and meta-analysis study, PNI had a negative correlation with disease severity in patients with COVID-19, and PNI was found as a predictor of mortality with a pooled sensitivity of 0.76 and specificity of 0.71. Besides, low PNI increased mortality risk seven times in hospitalized COVID-19 patients (27). While conducting our study, there was only one study in the literature comparing SII, GPS, and PNI in COVID-19, and this study revealed that only PNI was an independent predictor of in-hospital mortality of COVID-19 (28). Therefore, we included SI, GPS, and SII in addition to PNI in our study that aims to explore their role in predicting mortality in COVID-19. Consistent with the last-cited study, we found PNI to be the only inflammation-based prognostic risk score for predicting in-hospital mortality, with a sensitivity of 80% and a specificity of 64.1% at an optimal cutoff value of 40.71.
The PNI score can be calculated easily and quickly from routine blood tests in every patient suspected of COVID-19. Therefore, it is reasonable to use PNI to estimate mortality and the need for intensive care when variants of COVID-19 continue spreading worldwide and remain deadly. In addition to markers such as PNI, laboratory findings and the other risk factors associated with mortality or severe disease are potentially vital for the early detection of critical illness, provision of medical treatment, and improving prognosis.
This study has several limitations. Firstly, it is a retrospective single-center study with a relatively small sample size. Secondly, prospective follow-up and examination of the patients were not performed to predict long-term mortality risk; more extensive prospective studies are needed. Thirdly, including PCR-negative but tomography-positive patients in COVID-19 may cause an unexplained gap in this study. Finally, only intensive care unit patients who were already at high risk for severe SARS-CoV-2 infection were included in the study, which may be the major limitation of our study.
In our study, PNI was the only independent predictor of in-hospital mortality in COVID-19 patients among these inflammation-based prognostic scores. Hence, the PNI was a simple and applicable tool for estimating in-hospital mortality in COVID-19 patients. However, the role of PNI in short-term and long-term mortality among COVID-19 patients should be verified with further prospective randomized controlled studies.